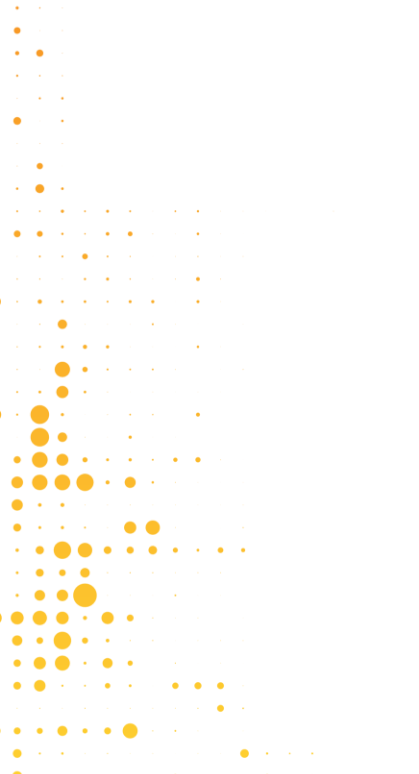
Discover more with a powerful, open science platform for biology
The Department of Energy Systems Biology Knowledgebase is a community-driven research platform for systems biology.
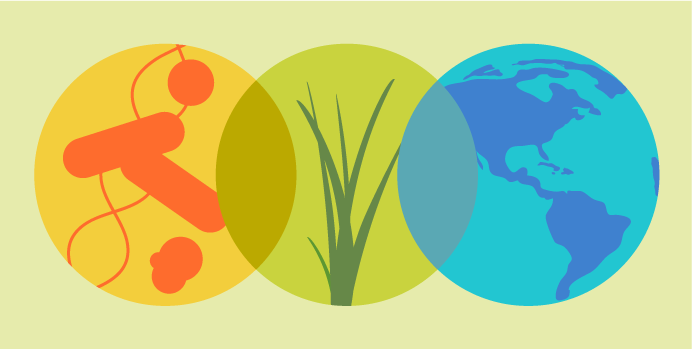
Create shareable, reproducible workflows in digital notebooks called Narratives with data, visualizations, and commentary.
Run powerful bioinformatics tools using freely available Department of Energy high-performance computing resources.

Use popular and interoperable open-source tools to enable a range of analytical pathways for your science.
Analyze your samples in the context of public data from the DOE and other public resources with privacy controls and provenance to track development of data.
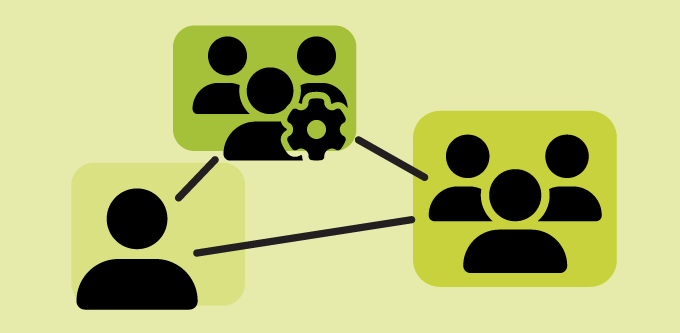
Share your data and analysis with your colleagues or the broader scientific community with publishable and FAIR Narratives.
Create and build organizations to manage data access and sharing across your project, laboratory, or institution.
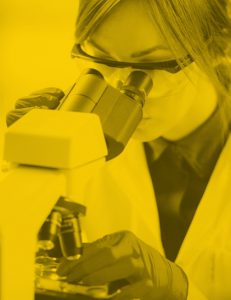
KBase Partner Institutions
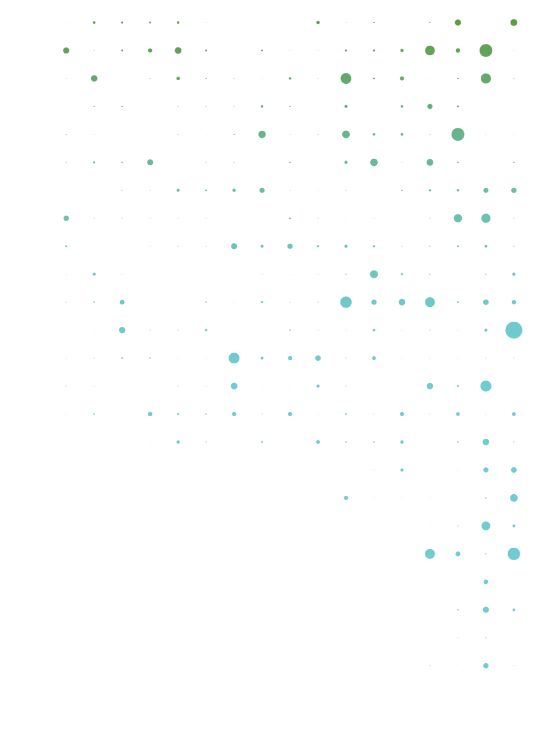
Looking for information on tools and resources?
Check out KBase Documentation for our Getting Started guide and information on tools in the App Catalog. KBase is a fully open source software project available on GitHub.